Client
The client is a 66-year-old Japanese automobile giant, recognized globally for its diverse portfolio of products and services. The company combines product sales, service, and coordination functions worldwide, excelling in the distribution, marketing, and sales of automobiles, motorcycles, scooters, and all-terrain vehicles, power equipment products like garden tools and generators, engines products and marine engines, and aircraft.
To enhance operational efficiency and customer satisfaction, LTIMindtree collaborated with the client to streamline the process of identifying wear and automating the service experience. This initiative reduced customer complaints and empowered business stakeholders with better operational control, exemplifying the potential of AI in manufacturing to drive business transformation.
Market Dynamics
The increasing complexity of vehicle servicing and maintenance, combined with rising customer expectations for quick and accurate defect resolution, presents significant challenges for service centers. Traditional manual processes often fall short in handling large inspection volumes while maintaining accuracy, leading to inefficiencies and higher operational costs. Inconsistent image quality further compromises defect detection accuracy, resulting in delays and costly errors. Additionally, service centers rely heavily on labor-intensive manual processes that hinder efficiency and face challenges with rigid detection systems lacking adaptability for continuous improvement.
To overcome these hurdles, innovations in the manufacturing industry, such as AI-driven solutions, offer a transformative pathway. By streamlining defect detection, automating repair recommendations, and reducing manual intervention, these technologies enable manufacturers to enhance operational efficiency. As the manufacturing landscape increasingly prioritizes efficiency and innovation, adopting AI-powered solutions becomes essential for maintaining competitiveness.
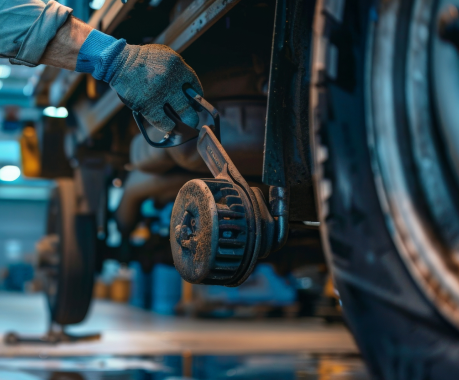
Our Approach
We developed a solution to streamline bearing wear identification and suggest methods for repairing. The process combines computer vision, deep learning AI models, and statistical approaches, reducing human intervention, all central to advancing AI in manufacturing.
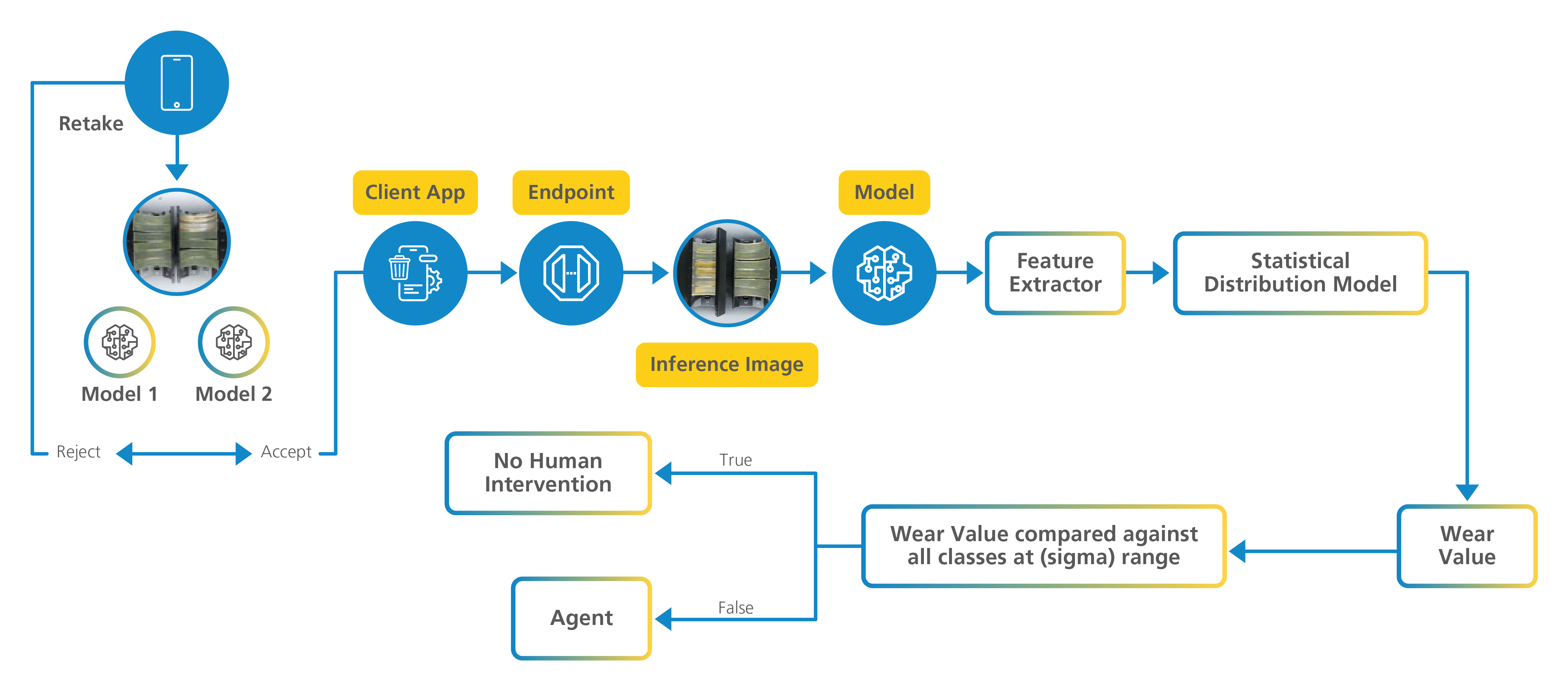
- Automated pre-screening: AI models inspect images to automatically classify them as ‘Good’ or ‘Bad’ quality.
- Auto defect detection: AI models detect defects and classify the type of bearing wear
- Continuous improvement: The model learns from defect patterns, improving detection over time and reducing reliance on manual intervention.
Solution Highlights
Working as a consulting and engineering partner, we guided the client through their transformation journey. Key steps included:
-
Discovery workshop
We identified user journeys and pain points in identifying bearing wear and repair methods at service centers.
Read More -
Application design
We created mobile and web applications for key personas: Mobile app: Captures bearing images and analyzes them. Web app: Displays analyzed images with AI-generated repair recommendations.
Read More -
Platform development
We built workflow management, search filters, overlay pop-ups, and report generation with email alerts.
Read More -
ML-Ops
We enhanced performance and scalability with model accuracy and monitoring.
Read More -
AI-enhanced inspection
Improved defect detection accuracy, enabling faster incident resolution.
Read More -
Statistical analysis
Continuous data analysis ensured high-quality AI model judgments, further reducing human intervention.
Read More -
Real-time updates
We provided real-time updates on complaint resolutions and ensured accurate data capture.
Read More -
Transparency and reporting
Continuous model improvement and monitoring ensured quick reporting and transparency.
Read More -
Proof of Concept (POC) for Enhanced Persona Experience Management
Read MoreWe developed early concepts for end-to-end wear identification, AI model selection, and outage reporting for stakeholders. This was enabled by: Design thinking Total experience strategy Image advisory Wear identification AI model ML-Ops Statistical analysis
Key Benefits
Highly robust detection model
Trained on approximately 30,000 image instances, our AI-powered solution ensures dependable and consistent performance across varied manufacturing scenarios. This capability underscores its significance in driving innovations in the manufacturing industry.
Superior accuracy
The solution delivers an impressive accuracy rate of over 85% for classifying bearing wear in real-world test environments. Image and orientation quality inspections achieve over 95% accuracy, ensuring precise defect detection and seamless operations.
Rapid inference capability
With an average processing time of just 1.5 seconds per image, the AI-driven system enables swift and efficient decision-making, streamlining critical manufacturing workflows. The platform's real-time updates and processing capabilities contribute to this achievement.
Continuous learning and improvement
By leveraging ongoing data integration, the detection model continues to evolve and adapt, maintaining peak performance while reducing the need for manual interventions. This approach underscores the transformative role of AI in manufacturing by driving operational efficiency and accuracy.
Conclusion
The transformation enabled by LTIMindtree helped the client streamline operations, boosting both user experience and efficiency. Faster defect resolutions, AI-driven process adoption, and a reduction in manual interventions led to increased customer satisfaction and operational reliability. To explore how our innovative AI and computer vision solutions can transform your operations, contact LTIMindtree’s technology solutions for Utilities here.
Looking to transform your operations with innovative technology and a persona-driven approach?