Operationalizing AI Ethics: Gaining Competitive Advantage for Global Acceptance
Organizations everywhere are adopting AI and machine learning in their systems, decision-making processes, and products. AI is being put in charge of many decisions which humans take cognitively — bank tellers, medical assistants identifying a disease based on symptoms, loan underwriters, language teachers, robo- wealth advisors, etc.
In his discussion on ‘Would life be better if robots did all the work?’ celebrated Harvard philosopher Michael Sandel debated whether AI automation would take away the self-worth and a sense of purpose that people get from their work or would it make life better by automating repetitive toil tasks and creating better opportunities? What if there was a universal basic income liberating people to pursue their passions in life? Would people be comfortable with robots taking their jobs? With an increase in cognitive capabilities and access to data from all over the world, would people start trusting AI more than a human doing certain simple and complex jobs?
In this blog, we will not be arguing whether it is ethical to adopt AI for certain jobs and its pace of adoption; however, we will talk about the aspect of AI programs operating in an ethical and fair manner.
AI systems learn and function through examples from training datasets. However, machines can learn in unexpected ways, and it is difficult to understand why they made a decision. Fairness, openness, equality of opportunity, and dignity for all citizens — can the new world driven by AI be good and just? Leaders of governments and private companies are keen on adopting AI with the intention of utilizing its benefits while not being clear about — what it means for an AI program to be ethical. Is it important to focus on fairness and transparency? Do AI Ethics pose any risk to them?
Though AI guidelines and frameworks are emerging, at the moment, they are very broad in context and difficult to translate into a specific action.
In order to build great systems and products using AI, it will be important to focus on AI Ethics.
The Mystery of black boxes:
Many machine-learning models are black boxes due to their complexities, such as ones using neural networks and gradient-boosting algorithms.
Interpretability means understanding a machine-learning model to know the important features and inner workings of the model.
Explainability means to make the model humanly understandable (users, business analysts, auditors, regulators) to answer the question of “Why” behind a decision. For example, an Explanation from a credit model — “This loan application was rejected due to FICO score and income features. If the FICO score is improved by 10 percent and income by USD 9k, the loan application can be approved.”
Some tools provide technical interpretation to help a data scientist tune the model performance.
So where is the issue?
In the case of complex models, interpretability is not easy. Even if the model is open source and we can read the code, we still cannot provide a complete understanding of the inner workings of the model.
Tools such as ‘feature importance’ provide effective interpretability for white-box models but fail on black-box models.
Complex models provide higher accuracy, and there is an increase in the adoption of these algorithms (deep learning/neural networks).
For corporates adopting black-box models, embracing explainability, transparency, and trust becomes a complex task.
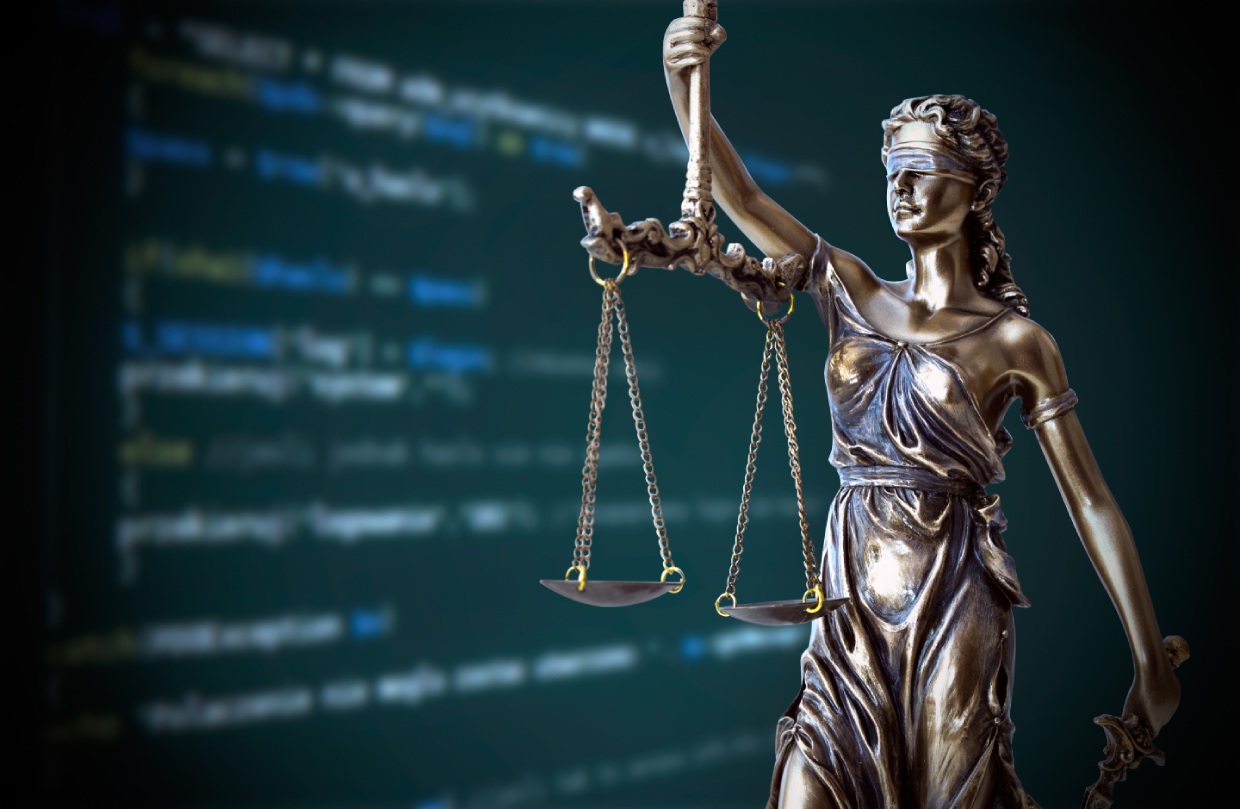
Pillars of AI Ethics are:
- Explainability
- Transparency
- Fairness
- Robustness
- Privacy
Risks
- Brand reputation: If there is a lack of trust, users won’t use the system, so it won’t matter how accurate it is. Social justice and fairness are top priorities for today’s consumers.
- Unexpected behavior: Many models have not been tested on real-world data. Testing has been performed on made-up data, not tested on real people. Current explainability techniques are still vulnerable and might be misleading, resulting in biased classifiers.
- Compliance: Regulatory and legal guidelines around data privacy, surveillance, and applications of AI are gaining rigor in modern governance frameworks. Compliance with these will be important for global acceptance. For example, GDPR, the EU’s Artificial Intelligence Act, and Australia’s AI Ethics framework.
Use Case — AI Ethics for Loans Underwriting
Banks are using AI for personalized digital services such as payment plans, investment advice, wealth management, credit underwriting, voice banking, chatbot customer services, etc.
Imagine that you have been assigned the task of creating an AI-driven digital bot to help with loan approvals and the underwriting processes. Here are a few questions you would need to ask,
- Is the training data sourced ethically? Consent must be taken explicitly from customers before the use of their profile data and lending history for training models. Implying consent might lead to a breach of data privacy laws and trust.
- Is PII and sensitive data stored securely? Regulations like GDPR and CCPA make it a legal obligation to protect PII data. Conduct a data discovery exercise to identify PII data spread across various databases and systems and use encryption techniques to secure users’ data. Not only for compliance or brand reputation, but data breaches can also have a significant financial impact on an enterprise.
- Is the AI doing good and working for the well-being of society? The system should be able to explain to users the reasons behind its decision. Example.1. Why a loan application was rejected, and what can be done to receive approval? This transparency will greatly help users to trust the bank and work towards making themselves eligible for loan in their next application. Example.2. Are good projects encouraged? Algorithms can be tuned to consider the carbon footprint and ESG score in order to fund environment-friendly projects and encourage organizations with good governance frameworks.
- Is the AI behavior consistent? In order to trust the results, its risk scores and loan defaulter predictions must be consistent for similar scenarios and inputs.
- Is the AI fair? The program should not be biased towards any group (gender/race/region/religion) where the loans are rejected or risk categorization/scoring is driven by personal and sensitive data. E.g., Equal treatment to new business loan requests from entrepreneurs irrespective of gender.
Operationalizing AI Ethics:
Corporates and financial institutions building and using AI products should consider the below steps to operationalize AI Ethics:
- Build awareness and credibility in users and investors on how the product complies with the ethics and moral values. AI applications have ethics embedded in the solution. With products like deep fake and data privacy breaches, profiling for political influence, and so on, people are becoming more sensitive about trusting AI applications and products.
- Conduct due diligence and risk assessment of the use cases to create categorizations such as “high-risk” “medium risk” and “low-risk”. Examples: “high risk” — autonomous vehicles, criminal justice, deep fakes, disease prediction using medical history; “medium risk” — financial advisor; “low risk” — Shopping recommendations. Companies need to ask themselves if the problem they are trying to solve necessarily needs to use AI. Can this technology be misused to harm society?
- Use XAI tools, as explainability and transparency go hand in hand with AI Ethics. A few of the popular explainability techniques are — LIME/SHAP/Protodash/CEM/OmniXAI. These help data scientists with the reason behind a prediction. However, the current techniques are still not entirely reliable and accurate.
- Invest in research and encourage open-source technologies to build new ways for explainability and fairness.
- Build a multi-disciplinary AI Ethics committee of stakeholders such as data scientists, Data and ML engineers, Business SMEs, Statisticians, Lawyers, and Philosophers/Ethicists. The responsibilities of this committee would be to create an AI Ethics standards framework and conduct internal audits to ensure fair and balanced datasets are being used and decisions are not biased towards any group, ensure data privacy and encryption, and ensure there is explainability and transparency so that trust can be built with users and comply with regulators, systematically capture the ethics related risks, and make management aware and avoid ethics washing.
Conclusion
AI-driven companies that embed their product within the strictest regulatory frameworks and build trust with users will have a competitive advantage with a wider global acceptance. Some key actions toward building an ethical AI would be to create awareness, form a committee, and diminish the risks that come with data bias, algorithm bias, privacy, legal compliance, and the trade-off between accuracy and explainability. It is imperative to look at the big picture to create a practical AI Ethics framework through which firms can function with human-like capabilities to empathize and act with moral responsibility.
Latest Blogs
In today's digital era, ransomware attacks and other cyber threats are more prevalent than…
In the evolving landscape of technology, the rise of quantum computing stands out as a frontier…
In contemporary corporate landscapes, the pursuit of human resources (HR) transformation remains…
In the dynamic realm of big data, advanced analytics, and artificial intelligence, the strategic…