From Knowns about AI, to the Unknowns about Operationalization of AI Models
The adoption of AI has invigorated businesses to look at new-age problems with a new perspective and solve them by leveraging the power of AI. That said, not all AI projects succeed. In a Gartner CIO survey conducted in 2018, whose findings are still relevant today, the research firm said that through 2022, 85% of AI projects would deliver erroneous outcomes due to bias in data, algorithms, or the teams responsible for managing them. In many cases, scaling the AI models into mainstream operations and moving AI models beyond the initial proof of concept and prototype to full-scale production remains a huge challenge. This is why despite the early adoption of AI by many organizations, few have managed to reap consistent business value from their AI investments.
With constant evolution in the type of data that is getting collected, there is a need for timely maintenance and monitoring of the model and operationalizing the models. It’s like any car we own; we need to maintain it properly and monitor its signals via telemetry. Similarly, when managing AI models, to scale them properly, we need to monitor the signals of the models and ensure that it is in the best of health for delivering business value. Simultaneously, other components of the data ecosystem, like feature engineering, model training, testing, and monitoring cohesively, are also equally important.
In a typical AI journey, we face a lot of challenges that hinder the prospect of model scalability. These challenges are typical in nature and range from the inability to show business value due to a lack of ground truth evaluation parameters to data infrastructure issues resulting in an inability to manage data efficiently. The results of a lack of a drift mechanism in unmonitored models leads to a lack of information in managing the model output. This can also cause issues with respect to model governance, serviceability, and deployment.
The end result being in this juggernaut, the models fail to scale, and these manual interventions increases the time-to-market. Now, with cloud computing becoming the new normal by 2024, it is expected that almost 70 percent of all the AI models will be cloud-based; the need is to have an efficient mechanism of scaling AI models.
There is a need for a holistic framework for operationalizing the AI models and bringing together the various key technology-enabled components under one umbrella, which is bundled with a people and process-enabled target operating model.
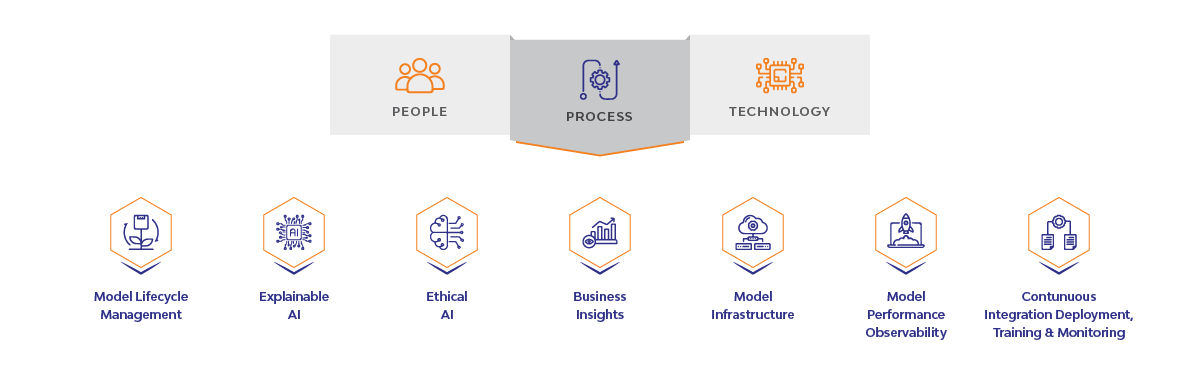
The focus must be on having a centralized mechanism to standardize and streamline the overall AI/ML model lifecycle and help organizations to achieve time-to-market and scalability by providing:
- An end-to-end model life cycle management including experimentation, training, deployment, and serving of the models across all key cloud hyperscalers
- The capability to ensure Infrastructure as a code by automating resource provisioning for training, scoring, etc., leveraging pre-built pipelines for the models
- The ability to decipher insights related to model explanations and importance of features for a particular model and bring in a responsible AI practice
- A centralized view of all models in the production to help monitor model drift, data drift, service health, along with a re-training mechanism
- A cohesive governance mechanism for the models wrapped with persona-based access mechanism and adherence to well-defined guidelines principles of responsible AI
- An automated model comparison and registry by extending DevOps pipelines, along with continuous re-training mechanism for the models
- The capability to attain business insights on the models deployed by leveraging key business metrics to capture performance and RoI on the models
Bundling all these key elements into a pre-defined target operating model will strategically enable business and AI teams to collaborate in a frictionless environment, which will ensure that the models can scale seamlessly from proof of concept to full scale production.
Latest Blogs
The business world is moving quickly and the only way to make informed decisions is to leverage…
As businesses turn to cloud services to meet their growing technology needs, the promise of…
Clinical trials are at the heart of drug development, producing vast, complex datasets that…
The rise of machine customers introduces essential questions that stretch our technological…